Posted By: Kylee Spencer, PhD, Assistant Editor, AJHG
Each month, the editors of The American Journal of Human Genetics interview an author of a recently published paper. This month we check in with Samuli (@samrip) to discuss his recent paper “Systematic comparison of family history and polygenic risk across 24 common diseases”.
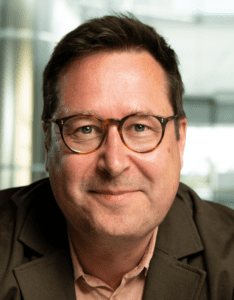
KS: What prompted you to start working on this project?
SR: When discussing the potential clinical utility of polygenic risk scores, a typical question from the audience is, “Why bother testing your genetic risk profile when the role of genetic risk can simply be measured using a simple question about family history of the disease?” In FinnGen data, we had 40,000 unique first-degree relative pairs as well as linkage to parental causes of death information, so that we were able to quantify how much overlap there is in predictive power between family history and polygenic risk scores (PRS) for 24 diseases where genetic risk factors are known.
KS: What about this paper/project most excites you?
SR: While there have been multiple papers looking at the question for individual diseases such as coronary artery disease, the prospective setup in FinnGen allowed us to create a comprehensive catalogue of joint effects of family history and PRS. From our results, it is clear that the two risk factors are complementary in all studied diseases. On average, the effect size for family history attenuated 10% when adjusted for PRS, and the PRS effect attenuated only 3% when adjusted for family history. This allows us to use the two measures together when estimating the risk for an individual. As an example, an individual with a family history of type 2 diabetes has a cumulative incidence of around 40% by the age of 80. However, if he/she is also in the top 10% of PRS, the cumulative incidence is around 70%. On the other hand, if the same individual is in the bottom 10%, the incidence in only around 20%. So, not all individuals with family history are the same. In other words, family history and PRS are not interchangeable measures of inherited disease susceptibility.
KS: Thinking about the bigger picture, what implications do you see from this work for the larger human genetics community?
SR: These two measures are easily available for risk estimation across diseases. For accurate risk evaluation of familial risk, using only one of them is not enough and together they provide a comprehensive view of a personalized risk in many diseases. When available, like in cardio-metabolic diseases, the risk may then be complemented with traditional risk factors and biomarkers.
KS: What advice do you have for trainees/young scientists?
SR: Get a broad knowledge of data science methods and their applications, also outside the field of statistical genetics. These will be your key tools for human genetics research. There’s now much more genetic data than there are capable data analysts, so no need to doodle with underpowered studies! Find a center with large genetic data resources and learn the details of the clinical and phenotypic data. This will help you to focus on the most important questions. Be ambitious and find a good mentor or two. Mentors can be from your institute, but also from collaborating institutes or elsewhere. Travel and see the world, visit different labs to see how they are operating and discuss with peers and colleagues in conferences and meetings over dinner or a drink.
KS: And for fun, tell us something about your life outside of the lab.
SR: Like many in Finland, we have a summer place by a lake where the different generations in the family gather to enjoy nature, pick mushrooms, grill, bathe in a sauna and dip into the lake, throughout the year.